In Technology We Trust
In Technology we Trust: How Can your Company Ensure its Tech is Ethical?
Listen to the blog here:
As the global pace of digitalization accelerates, businesses are becoming ever more immersed in technology, regardless of sector or core activity. But the rush to establish a use case and demonstrate the Return on Investment (ROI) has tempted many to overlook the ethical implications of technology.
But what exactly is ethical tech?
The World Economic Forum defines it as technology with a clear moral dimension. It should be designed and deployed with sufficient forethought to account for and promote human and corporate values, ethics and norms with fairness and transparency at their heart.
1. What benefits can ethical tech offer transitioning organizations?
First, it’s a golden opportunity to promote and safeguard a trustworthy reputation and avoid damage. It’s clear that there is a huge and proven potential of digitalization as a force for good within both business and society. However, mistakes happen as the field of Artificial Intelligence (AI), for instance, is fairly new and undefined, and many companies and institutions are still experimenting. These mistakes attract a lot of media attention, such as Amazon’s HR program that evaluated job applicants’ resumes to the detriment of women, or Facebook’s AI robots that had to be shut down after they started speaking to each other in their own language. Consequently, such cases raise general unease over the use of AI in decision-making and are cause for concern. Nonetheless, it’s important to state that these mistakes give a boost to development. It’s up to us to learn from them fast and establish improvements to build ethical tech in business and society.
Developing and embedding an ethical technology mindset in your organization when undergoing a digital transformation is crucial, since the consequences of an ethical tech incident can be substantial. With evolving digital technologies, ethical standpoints must evolve, too. What effect does it have on your customers, partners, shareholders, and employees? In the long term, an ethical tech company can enjoy competitive advantages by the chance to earn the trust of stakeholders by promoting human values.
How can you embed ethical tech into your digital transformation from the very start?
2. Transparency with data
Conveying a clear message on how you use data, and why you use it, should be a priority for executives committed to transparent operations. With ever growing quantities of data collected and stored from an increasing number of devices and sensors, an organization’s capacity to interrogate and analyze that data to gain valuable business insights becomes more enhanced by the day.
For instance, consumers’ personal details are now routinely collected and combined with an array of information harvested from their digital footprint. This might include social media posts, the route driven to the office, the buying history, internet search histories, and so on. The importance of data should not be underestimated; with society claiming the right to shine a light inside business practices to confirm whether corporate values align with their own, proactively ensuring transparency in how data is used becomes a business priority. This is also true for companies operating in the B2B sector since stakeholders are increasingly interested in ethical tech in the entire supply chain.
How can you establish data transparency?
An organization’s approach must be guided by a moral and human compass. To become an ethical tech company, leaders first need to establish and publicize company values. These should be based on customers’ expectations of openness and consent; The guiding principles of the General Data Protection Regulation (GDPR) illustrate that for the European Union: lawfulness, fairness, transparency, integrity, and confidentiality. That gives some guidance for the use of new technologies involving data and AI to strengthen our democracies, give people a voice and make meaning out of data to create a better future. Second, it should be ensured that the way data is used aligns with the organization’s values.
The overarching imperative for digital organizations should be to work on building trust in the security of that data and promote transparency around its usage. And that starts with policy and procedure. That’s why it’s important to build a robust data usage. This involves categorizing data methodically and keeping close tabs on all employees who have access to it. Everybody can then be aligned around your company’s approach to accountability for data, how that data is used and how it’s protected.
As a crucial part of ethical tech, cybersecurity should be baked into your plans from the beginning, with digital transformation risk assessment undertaken as your project is being developed. It should feature at every stage and needs to be preventative, detective and defensive. This reflects the reality that security is a multi-faceted and on-going challenge, and one that responsible and ethical tech companies rely on to build trust in their use of data.
By gaining user trust, companies will be able to gather more and more data to enhance their specific offerings. Many argue that the real value for users and customers will be lifted when companies start collaborating more in ecosystems, rather than trying to reap small gains through acquiring more data than their competitors. However, moving from ego system to ecosystem doesn’t mean that you share your data indiscriminately with everyone. Ecosystem means that you share information only with trusted partners who have a common and aligned approach to collaboration, where it is clearly defined who contributes what to achieve a common goal – for the good of companies and its clients.
With society claiming the right to shine a light inside business practices to confirm whether corporate values align with their own, proactively ensuring transparency in how data is used becomes a business priority.
3. Respect stakeholder privacy
A commitment to an unambiguous approach to privacy will help to build stakeholder trust in the protection of data. Historically, big data has been characterized by a ‘catch-all’ approach to maximize data collection, allowing organizations to quantify an individual’s everyday life to the benefit of the company collecting the data. Because organizations have been keen to exhaust all its inherent value, it’s not been uncommon to sell on or share customer data in a secondary market.
But most customers don’t fully understand the specific purposes behind collecting and sharing personal data. A fear that many users have is that their information is accessed by unknown parties and that their everyday choices are being tracked, ultimately undermining their trust in the privacy of their data. Though being mostly illegal but hard to trace, algorithms designed to influence political opinion, or to promote “fake news”, are presenting us with ever more dangerous applications that have the potential to erode privacy.
How can you establish stakeholder privacy?
Go out of your way to demonstrate good behavior and compliance, and actively seek to banish opaque policies that technically tell the truth, but don’t quite tell the whole story. Carte-blanche to use data as you like is no longer acceptable, and if your organization has plans to sell personal data onto third parties, your customers need to understand that in full, actively give their permission, and be prompted to grant that permission at regular intervals.
Giving stakeholders control over their data builds transparency and aligns your organization’s everyday activities with the values it outwardly promotes. This is already a legal requirement in some jurisdictions; in the EU, for example, the GDPR outlaws any processing or use of data that an individual has not given consent for. In fact, it explicitly states that “data subjects” can withdraw consent whenever they want, and those who hold the data are obliged to comply.
4. Build trust in technology
A variety of more sophisticated technologies, including AI and machine learning (ML), continue to move into everyday life. According to The Harvard Gazette, global corporate spending on AI software and platforms is expected to top USD 100bn by 2024.
In addition to some of its most visible and benign uses in buying recommendations on websites or in translation tools, AI is also already used in a wide array of more critical scenarios, from helping medical diagnoses and assessing mortgage applications to keeping driverless cars safe. As a result, human oversight is instrumental, not just to ensure that disaster can be avoided in the event of technology breakdown, but to act as ultimate arbiter of a technology that is still likely to produce further ethical dilemmas. In short, ethical tech requires humans to be responsible and accountable for the final decision, not AI. Complex decisions should be more transparent, with the roles of AI and human intervention clearly visible in the process.
How can you establish technology trust?
Organizations must establish processes to ensure that they and their stakeholders know exactly what technology is being used, where it’s being used and how. Creating trust among clients, partners and employees is dependent on ensuring methodical and consistent human oversight of critical systems.
That’s why you should encode your organization’s values. Digital technology can be developed to take account of biased training data which results in algorithms and AI decisions so that the technology operates within the bounds of your company’s values. With so much riding on client and customer trust in the fairness and ethics of modern digital systems, encoding ethical values so that they can be assessed and measured against technological business operations will help bridge the potential trust gap.
One way around developed biases is to explain how AI decisions are made. For example, with medical diagnoses that rely on AI, healthcare companies have examined ways to give each diagnosis a confidence score based on a variety of contributory medical and lifestyle factors, allowing clinical staff to follow the workings of algorithms and introduce the benefit of their medical training and experience into that diagnosis if needed. AI is not replacing human beings, it’s enhancing them. But human beings are still in charge.
Taking it a step further, Explainable AI (XAI) is bringing further transparency to decision-making. Defined as a system that can explain its decisions, as well as the rationale for those decisions and some notion of how it might behave in the future, the self-learning AI platforms that power driver-less cars, for example, now have the ability to “explain” their choices. In this case, choices such as changing lane or accelerating or braking for no apparent purpose – and how factors influencing those decisions – are weighted. Yet, research on XAI is still fairly young and many questions remain unanswered, leaving applicants of AI with the sometimes tricky trade-off between the performance benefits of an unexplainable state-of-the-art model and an explainable, but lower performing, commodity model.
Ultimately, leaders must decide how to put their company values into practice to guarantee stakeholder welfare through ethical tech. The global tech industry can only build trust by using technology in a responsible way that promotes transparency and a purpose to that technology that focuses on the good it can bring about for all of society.
5. Guide ethical tech from the top and from the bottom up
There is an opportunity for leaders to gain a significant competitive advantage by making trust a business-critical issue.
How can you establish this?
It’s equally important to drive ethical tech both from the top of your organization, and from the bottom up, too. Leaders need to communicate their company’s values and ethics, formalize them in policies and make sure technology use is aligned with those values. That process can be set in motion by making sure employees understand the commitment and dedication to ethical technology. When leaders communicate that clearly, it helps others throughout the company to buy into that sense of responsibility, and will inform ethical decisions aligned with company values.
Digital technology use cases that are specific to your company can help employees test your decision-making framework against real-life scenarios, and that framework can be used to question and adapt the way individual employees make responsible decisions. In turn, employees are then more disposed to develop a natural ethical mindset of their own, one that is more likely to manifest itself in all facets of their roles.
And that’s where the bottom-up approach comes in; by taking your cue from the Tech for Life movement – which establishes a “code of honor” for the tech community regardless of a person’s status within a corporation – you can help foster this bottom-up movement in the direction of socially responsible tech based on a human moral compass. After all, although it needs to be guided from the top in the design stage, ethical tech is a responsibility shared between each and every one of your employees across all functions.
Remember, data regulations exist to enforce broad minimum standards of protection; for example, the EU’s GDPR can penalize data misuse and breaches with fines of between 2% and 4% of worldwide annual revenue, or flat fines of EUR 10m to EUR 20m respectively, whichever is higher. Similarly, for the use of AI, the EU is acting as a driving force to establish legislation and we can expect laws and regulations to come soon. But if ethical tech comes from the bottom up and from the top with conviction, those values and ethics will infuse every decision with responsible ethical corporate values, policies and procedures that guarantee even higher standards around data use.
From the bottom, from the start & from the top
Ethical safeguards, policies and procedures can only go so far. To truly master the art of building trust in a burgeoning tech landscape, you need to devise ways to equip employees with a mindset sensitive towards ethical standards. The role of leaders today is to embed those values into the culture of their organization, from the bottom, up and from the top, down. That way, you’ll gain trust in how you use and collect data, in how your technology is deployed to make decisions, and in the privacy of all stakeholders; top-down and bottom-up leadership will ultimately help weave an ethical technology mindset through the fabric of your digital transformation to prioritize the relationship between technology and human values. Everyone involved in tech should apply human principles guided by an ethical compass to ensure that tech is used in a responsible way.
So, set out today to make sure your organization is becoming an ethical tech company in parallel to embarking on your digital transformation; start at the planning stage, apply ethical tech standards iteratively depending on your industry and business, and your efforts can offer a competitive advantage in the form of the trust of stakeholders, clients, and investors alike.
Free whitepaper
Download our whitepaper and learn how to develop comprehensive data strategies to identify new business opportunities and prepare your company for the digital age!
Our expert
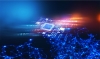
The Route to Connected Vehicles of the Future
The Route to Connected Vehicles of the Future: How Edge & Cloud Ecosystems are Paving the Way
Listen to the blog here:
The number of connected vehicles around the world is growing rapidly. It's estimated that by 2025, when 100% of all new vehicles to market will be connected, there will be more than 400 million connected passenger vehicles alone. More connected cars require connected vehicle ecosystems in smart cities to function efficiently. The market is expected to see a compound annual growth rate (CAGR) of 17% between 2019 and 2027, amid increasing population density in urban areas. According to the UN, nearly 70% of the world’s population will live in cities by 2050. So, the performance of modern networks to support connectivity is becoming even more critical.
Whether automotive industry players will be successful or not depends on how they leverage ecosystems and combine technologies like edge and cloud computing. In tandem, they can deliver a wealth of benefits, including safe and reliable autonomous driving, energy optimization and real-time navigation, as well as new data and subscription services.
To pave the way for connected vehicle ecosystems, read on!
1. Why connected vehicles and their ecosystems will fuel the future
So what, exactly, is a connected vehicle? It’s one that can be linked to different services and devices via wireless networks. This includes other connected car technology such as software, entertainment and communications, or parts of infrastructure such as traffic signals, emergency centers and navigation aids, as well as other vehicles, and even bicycles, cyclists and pedestrians.
A connected vehicle ecosystem refers to everything that will connect to the car via smart-city platforms. It’s the sum of every fixed or mobile device or sensor that can connect to tomorrow’s cars. It will be able to collect and process information on everything from location, weather and driving conditions, to parking availability, congestion or hazards ahead.
In short, both will become part of tomorrow’s inner-city networking with a seamless and continuous exchange of information and data via fixed devices, sensors, and via the edge and automotive cloud services.
As 5G grows, and eventually gives way to 6G a decade or so later, those connected vehicle ecosystems will be key to providing state-of-the-art vehicles with the ability to become sentient machines. They will rely on the transfer of data through wireless communications for everything from situational awareness to predictive maintenance.
How will this benefit users?
Broadly speaking, the term “users” will include not just passengers: fleet owners, smart cities, original equipment manufacturers (OEMs) and original equipment suppliers (OESs) will all stand to gain, too.
Connected vehicle technology will offer services including driver assistance, safety features, entertainment, well-being, as well as vehicle and mobility management. Vehicles could receive rapid product iterations over-the-air to further improve software functions. Users will be automatically connected to ecosystems to enable easier road charging and reactive traffic monitoring and management – improving the driving experience significantly.
For businesses, connected vehicle technology will also open up the potential for monetizing connected-car data with big data analytics. Connected vehicle ecosystems will also soon enable the wide-spread use of fully autonomous vehicles and will offer predictive maintenance and decentralized fleet-management features, while significantly improving vehicle condition monitoring, predictive analytics and optimized fuel/hydrogen/electricity consumption. From a strategic perspective, many of these benefits will ultimately aid a gradual move towards a vehicle-as-a-service model, where subscription models based on usage rather than ownership gain market share.
The potential for businesses and passengers alike to unlock the future of mobility is clear. But how to untap it?
2. Edge / cloud and IoT platforms can be key enablers for connected vehicles
The automotive sector is awash with buzzwords relating either to the edge or to the cloud. So far, key players have tended to focus on just one of these technologies. The sweet spot, however, is in the combination of both edge and cloud: each will need to work together to truly enable connected vehicles.
Despite an on-going and increasing shift to the cloud, pushing everything to the cloud is expensive. And although current cloud connectivity cannot, without latency, support uploading and processing the 1 GB of data that connected cars will generate every second, the ability to do that isn’t far away.
So, from a strategic perspective, when many functionalities can already be handled on the edge, the key question for automotive players is: which particular service categories warrant the cloud and which can be processed on the edge?
Automotive edge computing involves data processing and analytics physically close to where data is generated and collected. At its simplest, it narrows the gap between data storage and the devices that rely on it to function within a pre-determined timescale. This means that latency problems can be resolved by meeting the sub-1 millisecond reaction time that safety-critical features demand. Not only is it more dynamic and flexible than the cloud for certain applications; for many service categories, it’s also more cost-effective. When combined with IoT technology, edge computing saves bandwidth, allowing you to allocate resources efficiently.
When deciding between edge and cloud solutions, the crux lies in differentiating between service categories to make those decisions more manageable!
3. Developing your combined edge-cloud ecosystem nails down to two decisions!
Seeing the car as part of the greater ecosystem is key, and building your ecosystem boils down to two issues:
- Which partners do you include?
- How do you combine both technologies to focus on the sweet spot?
Choose your ecosystem partners well:
Many providers claim expertise in either edge or cloud technology, but choosing the right partner is not as easy as it seems. Include your entire value chain – from the very beginning, think about hardware and software providers, developers, national government organizations, OEMs, OESs and beyond. After doing so, it’s crucial to pull the network together by fostering continuous interactions, enable seamless interaction based on an open technology architecture and utilize joint data to continuously maximize potentials of the collaboration within the ecosystem.
Decide where to combine edge and cloud technology:
Which vehicle features should be in the cloud and what makes sense on edge? The crux lies in differentiating between service categories to make those decisions more manageable. Driving assistance such as collision avoidance, for example, is currently better suited to automotive edge computing, where a low latency, or fast reaction time, is needed. The edge also supports other safety-critical features such as lane discipline or traffic-sign recognition. Furthermore, vehicle-to-vehicle (V2V) and vehicle-to-everything (V2X) communication via Dedicated Short Range Communication (DSRC) and Cellular-V2X (C-V2X) can be used to transmit e.g., the previously detected red traffic light to another vehicle. Navigation, on the other hand, can be dealt with by automotive cloud solutions, as can features such as energy efficiency, vehicle security and multi-level authentication. In addition, some subscription and premium data services may warrant the higher costs of cloud. In short, automotive cloud services are suitable for use cases in which higher latency is acceptable or desirable, and where subscription revenue streams warrant the cloud.
How you maximize the benefits of each technology, once safety-critical features are assured, ultimately comes down to a strategic choice: which applications and services justify the higher costs of the cloud, and which ones can save bandwidth on the edge?
4. Summary – more than the sum of its parts
The key to developing an edge-cloud ecosystem is to view vehicles as an integral and integrated part of a much bigger system. A connected car is connected to a fleet, which is in turn connected to entire smart-city platforms comprising everything from highly dynamic and unpredictable road and city users to transient static features such as adverse weather, lane closures and accidents.
With demand for smart-city ecosystems that encompass passenger vehicles and their connected vehicle platforms as part of the whole system, it’s crucial to build a partner ecosystem along the whole value chain. Prioritize the integration across the partners and focus on easy technical connectivity. Here, it doesn’t have to be an edge vs cloud decision: It’s crucial to find a way to combine them efficiently for each individual use case!
Free whitepaper
Read our whitepaper to learn how to develop comprehensive data strategies to identify new business opportunities and prepare your company for the digital age!
Our expert
Author
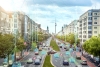
4 Challenges for IoT Implementation - And How to Overcome Them
4 Challenges for IoT Implementation - And How to Overcome Them
Listen to the blog here:
The transformative potential of IoT as an enabler of digitalization across all industries is increasingly seizing executives’ attention. However, our partner pulse survey with Harvard Business Review Analytic Services also identified certain challenges regarding IoT that potentially hinder business leaders to kick off their digital journey.
Although three quarters of respondents said they felt under some pressure to quantify and leverage the potential of IoT benefits before their competitors get there first, only 10% were able to measure the return on investment (ROI) on most of their initiatives. Nearly half were worried about change management challenges, 45% admitted to not knowing where to start with IoT, and a majority reported they were still trying to identify potential use cases.
With the underlying imperative that technology must serve business, people and our wider society, how should leaders overcome these specific hurdles to make their IoT initiative a resounding success?
Let’s have a closer look at the top four IoT adoption challenges.
1. ROI challenges – determining the impact of your initiatives
90% of executives struggle to accurately measure the return on investment (ROI) of their IoT initiatives. This is one of many common IoT challenges and issues in large and complex organizations that raise executives’ doubts in the impact and value of IoT for their businesses.
The return on investment is defined “simply” by the ratio between profit and the cost of the investment based on discounted cash-flow calculations. However, it is still a huge roadblock for leaders to accurately measure and calculate it.
How to overcome this?
The first thing to remember is that leaders should only consider starting their digitalization and IoT journey once they have established a clear business goal. Companies tend to “fall in love” with a new technology but forget about the actual business value it should bring to them. The real value of digital initiatives can be seen and assessed more clearly if executives flip the question of ROI around: Instead of looking for the ROI of a specific IoT solution, they should be asking how to calculate the ROI when using IoT to solve a business problem and create a lasting impact. Only when defining the business goal of the initiative as precisely as possible, executives will be able to accurately measure whether it will pay off in the future. The way the solution solves the business problem defines the return of the initiative.
Therefore, the investment budget always needs to consider transition costs for change management, the adaptation of processes and tools and skill development next to technical costs.
Only when taking all of these aspects into consideration, the real return of your investment will become visible. More details about accurately calculating the ROI for IoT initiatives can be found here.
To view the Key Challenges for IoT graphic in full width please click here.
2. Change Management challenges – putting your people at the core
As pointed out earlier, one of the main causes of failure stems from a misconception of IoT as an initiative based primarily on new technology. In truth, IoT is a people business, and the success depends on taking the employees on the journey from the start. However, executives often don’t know how to prepare their employees for what is to come.
How to overcome this?
For employees and leaders alike, some of the most challenging aspects of IoT are characterized more by the need for a cultural transformation than a technological one – this requires a structured change management approach from the very beginning. First and foremost, an integrated approach across both tangible and non-tangible elements of the transformation is needed. That means bringing together technological and process elements with social factors such as corporate and team culture. Both aspects are equally important to create employee buy-in.
Additionally, an understanding and acceptance of evolving target states – part and parcel of any digital transformation – is crucial. Here, leaders need to foster an agile mindset. During the transformation, needs and overall objectives might change unexpectedly – that’s just natural. Flexible concepts and an agile approach are needed to constantly adapt to new requirements – while not losing people along the way.
In the context of IoT projects, classic change management levers – communication, leadership, team configuration and ongoing training – have to be adapted to the digital environment. One possible starting point for change management measures is to check the employees’ readiness for change in general and digital readiness in particular. This will help to identify the organizations change capabilities and address any gaps in digital core competencies and to prepare the workforce for new and changing job requirements.
To carry the digital mindset into the organization, the successful approach of one of our clients, a leading energy equipment supplier can be adopted: the client identified digital role models in their workforce to act as ambassadors and coaches. With full access to the digital transformation strategy, their role is to report back to colleagues and help lead them through the necessary changes, meaning nobody’s left behind. In our client’s case, it gave their engineers clarity on future roles and training, and resulted in their engineers proactively engaging in the successful launch of their AI design algorithm for their parts. However, this is only one specific action that can be taken to put your employees at the core of your digital transformation journey.
Connecting the OT and IT world brings along several unknown cyber challenges as the number, diversity and complexity of connections amplify.
3.Cybersecurity challenges – balancing cyber risks & fears with IoT benefits
Business leaders are naturally worried about the risk of cyberattacks. With more and more devices and assets connected and networked, executives are becoming more wary of an increased digital attack surface and greater vulnerability. IoT security challenges can range from customer data theft to ransomware to industrial espionage and are driven by increasing professionalization of cybercrime. As the number of vulnerabilities increases, the attack surface becomes more important to defend.
Also, connecting the OT and IT world brings along several unknown cyber challenges as the number, diversity and complexity of connections amplify: a secure linking with the cloud, the industrial control center or third-party providers need to be guaranteed while availability and connectivity of the assets need to be ensured at any time. In general, the discrepancy between the OT and IT network logic needs to be considered to define what are the challenges of IoT here.
Nearly half of respondents in our HBR study rank cybersecurity as an issue that merits close attention, and over one third report that it’s among their top concerns.
How to overcome this?
Step one is to understand that even if a company already has complex cybersecurity defenses in place, they usually only protect the IT world. In the IoT / OT landscape there are new problems which is why companies need to adapt their approach. A common pitfall is to threaten the IT landscape by neglecting the operational technology (OT) network. This brings additional risks which the companies need to mitigate.
Companies should use the planning phase to embed security into their IoT project and establish threat risks with a full IoT risk assessment while projects are still being developed. Therefore, a risk-based approach that prioritizes vulnerabilities and threats can be the answer: Starting with prevention is crucial to make it as difficult as possible for an adversarial to break into the systems. If the cybercriminal finds a way in, companies need to detect the threat actor as fast as possible.
Especially in the IoT environment, it is essential to have a risk-driven strategy in place and create transparency over assets. Cybersecurity departments need to make sure to know, manage and track all endpoints and devices during the entire lifecycle. Weaknesses need to be identified and therefore, effective threat monitoring solutions implemented. Applying new principles like “zero trust” to control and verify all actors in the network as well as every data stream can help to prevent disruptions before they occur.
4. Asset management challenges – connecting the dots between assets
By connecting assets with digital environments and data analytics, IoT can be a main enabler for asset monitoring and tracking, effective resource management and maintenance optimization – especially in the manufacturing environment. Measures of predictive maintenance can for instance prevent factories from unplanned production downtimes and therefore, can ensure production and supply chain functioning. At the same time, data-driven decision making can optimize maintenance schedules and improve investment decisions.
However, the big initial question is: how to actually realize the connection between operational (OT) and information technology (IT) – especially for aging, proprietary manufacturing assets? Due to this difficulty, this is often believed to be one of the main IoT implementation challenges as it requires greenfield sites which hardly exist in reality. This makes business leaders doubt the business potential of IoT. Also, IoT implementation leads to more connected devices in the ecosystem causing higher asset diversity, volume and in the end complexity – especially when trying to adapt many diverse IoT technologies at the same time. Localizing even more assets in real time might be challenging as well.
How to overcome this?
Companies should apply a step-by-step, structured approach: Sensors and connectivity should be added gradually to existing machinery, allowing to harvest data and gain valuable insights one machine at a time. This gradual implementation allows executives to optimize existing assets and control costs. A carefully tested and piloted path can equip machines with IoT capability causing only minimal impact on the production line. Doing so, executives can demonstrate the overlooked value of IoT, which lies in upgrading brownfield environments consisting of older equipment step by step. Sensor monitoring, data analytics and predictive maintenance, for example, can be deployed on legacy assets without widespread risk to the production lines.
To find the right sensors and data collectors, might even require a certain amount of creativity – especially in brownfield environments. Established machinery is often hard to connect to state-of-the-art sensor technology. For example, to obtain data from a shop floor with machines not originally designed to deliver operational data, a camera system monitoring the shop floor could provide valuable data that can be further processed and evaluated, thus creating a potentially valuable use case.
The complexity and diversity of data often requires major efforts in processing and evaluating the information generated from assets. To overcome this, implementation teams that include IT experts, domain experts, and data scientists are needed. Furthermore, in complex cases, no company can do this alone, so a co-creation between different partners of an ecosystem can be the answer.
After tackling these initial hurdles, the potentials of IoT for asset management are limitless: Optimizing the OEE (Overall Equipment Efficiency) makes it possible to not only manage the physics of assets, but even their usage itself. Track & Trace solutions can be the foundation of an e2e supply chain, connecting inter- and intra-logistic.
5. Four solutions and a world of opportunity
IoT has little value without an established business case, benefitting people and society. Here we’ve identified what are the main challenges of IoT faced by organizations and their potential solutions.
What they show is that a change in perspective of the way the ROI is calculated can reveal the true value of IoT; that the employees should not be treated as an afterthought; and that the machine assets should be digitalized gradually and creatively to maximize output whilst controlling costs and optimizing utilization. And all insulated by effective cybersecurity defenses to shield the data, assets and networks from attack while ensuring availability.
What executives can achieve by overcoming these hurdles presents an even greater prize than merely the sum of their parts: A resoundingly successful IoT project that generates benefit to the company and its stakeholders.
With the confidence to face the challenges head-on, executives are more likely now than ever to be able to seize the significant advantages of IoT to leverage these advantages ahead of their competitors.
Now’s the time to seize the significant opportunities that digitalization offers.
FREE HBR PULSE SURVEY
Few emerging technologies offer more transformative potential for forward-thinking companies than the internet of things (IoT). The reason: IoT combines sensors and sophisticated software analytics to process large volumes of operational data. Download the study for a moderated introduction to IoT.
Our expert
Author
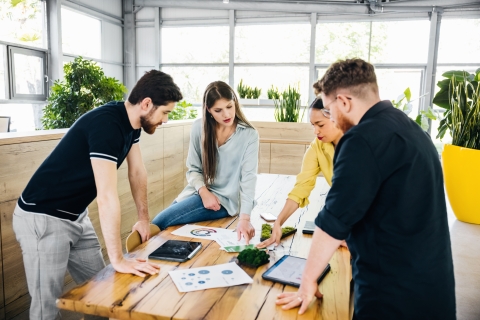
Digital Twins to Decarbonize Energy Systems
6 Success Factors for Using Digital Twins to Decarbonize Energy Systems
Listen to the blog here:
Let's face it – the world needs to overcome a big challenge with regards to energy: After all, the energy consumption of cities and industries is ever-growing. According to World Bank data from 2019, the proportion of the global population that resides outside of cities and towns has fallen by two-thirds compared to the start of the 1960s. At the same time, academic studies have noted that urban areas account for about three-quarters of global energy use, something that will undoubtedly cause a problem for ongoing sustainability efforts.
Even with more and more green energy sources coming online with every passing month, there is still an extensive challenge to lower carbon dioxide levels in the atmosphere and for the nations of the world to meet their Paris Accord climate change commitments. Many businesses have committed themselves to lowering their carbon footprints to meet regulations and cover new customer demands. Siemens, for instance, was actually the first DAX company to commit on carbon neutrality by 2030 and reduced emissions already by 54% since the start of the program. Consumers increasingly turn to companies that focus on sustainable energy generation and consumption and realize that a profitable future is only possible in a sustainable world. Either way, I already see a world in which decarbonization is considered to be of huge importance in the urban realm. However, many companies still lack a holistic approach to decarbonize their energy systems.
In this article, I will emphasize the relevance of decarbonization for the energy sector and the related energy-intensive industries as well as for sustainable urbanity. Then let me show why digital twins should have a part to play in any decarbonization roadmap. Finally, I will share six identified principal success factors on how to apply digital twins to decarbonize energy systems.
1. Energy is at the heart of the green revolution
Sustainable energy use is at the heart of the fight against climate change, as energy generation is responsible for a huge amount of carbon dioxide (CO2) and other greenhouse gases that get emitted into the atmosphere each year. Only for the purpose of illustration, according to the Energy Information Administration (EIA), electrical generation accounted for some 4.13 trillion kilowatt-hours (kWh) of energy in 2019 in the United States alone. The EIA estimates that some 1.72 billion metric tons of CO2 was released into the atmosphere as a result of all energy sources. To put it simply, that's approximately 0.92 pounds of CO2 emissions per kWh!
Numerous decarbonization trends, such as the switch to biofuels from fossil-derived ones, already try to tackle that problem today. Others include the increasing use of wind energy, both on and off-shore, as well as the development of hydrogen-based fuel cells, or the roll-out of carbon capture and storage (CCS) technologies. These truly are groundbreaking times for decarbonization!
Nevertheless, significant quantities of greenhouse gases are still being produced every day due to “business as usual” operation of energy systems in business, housing and smart life, not to mention the mobility and transportation sectors in cities. The good news is we can keep a cap on these emissions. With the fundamental optimization of the entire energy system to known decarbonization solutions, the world will come closer to the goal of meeting its climate agreements and lower cost pressures to bear on climate goals. This is especially the case with energy systems that offer huge potentials for CO2 reductions. Energy systems are basically interconnected networks that deliver energy to end users – both industry and consumers – on an on-demand basis. Sometimes demand may be high and sometimes it may be low, that’s just natural. Any energy system must account for this and be run in an optimized way so that over-generation of electrical energy is minimized or even avoided. In this regard, digital twins represent a powerful ally in modern decarbonization technology today bringing together two major goals for businesses: sustainability and cost effectiveness.
To view the Digital Decarbonization graphic in full width please click here.
2. Digital twins can help to decarbonize energy systems
There are several technologies that already exist which contribute to the reduction of CO2 – and other greenhouses gases – from being released into the earth's atmosphere. Among these technologies, digital twins can be a way to bring all technologies together and model energy flows and changes of parameters in real time. Simply put, a digital twin is a virtual version of something that usually resides in the physical realm and is used to mimic real systems or products in industrial environments. It was once used to help designers and engineers with new product concepts but, strengthened by the Internet of Things (IoT) and Artificial Intelligence (AI) systems, it has proven itself to be an even more valuable tool. This is because it can model a raft of interconnected systems through big data analytics.
In general, one can now model energy systems and infrastructures within smart districts and utilities – and even across entire national networks, if up-scaled – by creating digital twins of the as-installed or as-planned grids. The basic idea is that digital twins help with the decarbonization of energy systems by mapping all of its aspects – from electrical production to distribution losses and localized demand – in the digital realm. It is already proving itself to be particularly effective in energy management as well as district heating, electrically powered public transport infrastructure management, and sector coupling, to name just a few areas. Therefore, it is important to know which factors in the deployment of digital twins will lead to the most successful outcomes. I identified the top six success factors to successfully decarbonize energy systems using digital twins.
Digital twins represent a powerful ally in modern decarbonization technology today bringing together two major goals for businesses: sustainability and cost effectiveness.
3. 6 success factors for using digital twins to decarbonize energy systems
1 - Don’t start without a decarbonization strategy in place
To begin with, any successful decarbonization program must commence with clear goal-setting. The modeling used in the digital twin of the effective electrical system should offer a clear decarbonization roadmap with carefully thought-through criteria about the intended outcomes. First and foremost, the monetization of the current versus the targeted carbon footprint comes into play. Also, current and future business models focusing on either private or public goods or services will get conceptually challenged based on their inherent carbon intensity. Subsequently, the question of the future energy mix along the entire value chain of production or servicing has to fully include all suppliers and business partners – and this can even raise new questions related to the own location strategy. Finally, sustainability reporting should not be underestimated, as it even exposes management to personal liability.
2 - Always consider the whole system!
Secondly, you always need to consider the entire energy system when starting your journey and how to best optimize it for favorable outcomes. It regularly includes electricity, but also heating & cooling and transportation for a thorough end-to-end analysis of the initial situation and therefore the entire technological framework conditions. To name only a few, each site or entity calls for a multidimensional consideration of e.g., technical lifetime, maximum capacity, minimum versus maximum operating and down time, investment and maintenance cost, and of course its energy demand and relative efficiency. In other words, this attention to detail and completeness is needed to unlock the full decarbonization potential.
3 - A technology-neutral approach is vital
Having a technology-neutral approach to the decarbonization of energy related systems in place means that you should have no preconceptions about which model or configuration will work when adopting digital twin technologies. You need to start the journey with an open mind. A technology-neutral approach allows to draw flexibly from a variety of existing ideas and possible technology solutions. In short, predetermined technological pathways tend to end up with sub-optimal outcomes. Never commit too early to a single technological approach when examining all of the conceivable approaches to decarbonization because your digital modeling and AI number-crunching may surprise you!
4 - Data is the new gold
Preparing your dataset before you build your digital model(s) is something I have learned first-hand to be crucial to the entire process. In short, this means gathering, cleaning and structuring the data, but it will also require that the plausibility of the required data in real-world settings is checked. If you put garbage data into your digital twin model, then expect low-quality outputs. All too often, we have experienced these challenges when analyzing for example meteorological data being crucial for renewable solutions next to data sets about electrical consumption in buildings or feed-in profiles from photovoltaic, solar thermal, concentrated solar, wind, and hydro power plants at locations worldwide. In general, some patience paired with professional experience is needed to find, prepare and make the best use out of your data. There can never be enough data preparation and checking!
5 - Take your time!
Since digital decarbonization is obviously such a highly multi-faceted approach, better avoid looking for quick wins. Preparation of the energy system model needs care because it will be more complex – often much more complex – than you had initially bargained for. For sure, it all depends on the size of the endeavour: it takes rather a few months than weeks when adding renewables into an existing fossil power generation system as this might require spatial decoupling of power generation and consumption to handle imminent grid constraints and finally, a flexible use of battery storage systems. It might also take some time to onboard the right experts especially the ones with field expertise – but believe me, it’s worth it. In general: Success doesn’t come overnight, after all!
6 - Know your technology well
Although the decarbonization of energy systems obviously requires know-how with electrical gerneration, supply, and distribution, the final factor I recommend prioritizing if you want to achieve successful decarbonization is expertise with digital (twin) technology itself. Put simply, you require a high-level understanding of data modeling as well as expertise in smart data technologies if you want digital twins to help decarbonize your energy system. If you don't possess these skills in-house, then prioritize outsourcing them before starting your decarbonization journey. Digital decarbonization always need these most experienced practitioners coming from multiple disciplines to optimize the specific decarbonization path.
4. How digital decarbonization can change the world
When applying a strategical approach, the impact of digital decarbonization can be quite impressive in numbers: for instance, in one of our projects with a German city with about 200,000 residents, we modeled its energy demand and infrastructure and found that 70 percent fewer emissions by 2035 would be perfectly feasible. This project also demonstrated that a 25 percent reduction in heat demand could be achieved in the same period which is a fantastic result by any standards.
In general, the main advantages of utilizing digital twins in future decarbonization strategies will be felt in basically all public and private sectors. Given that buildings account for about 40 percent of all current carbon emissions, focusing on the digital decarbonization of energy systems will make a huge difference to the climate of tomorrow. While decarbonization resonates best with the utilities sector as a whole, high energy demanding sectors such as the chemical and similar process industries, deserve highest attention as well. Benefits like running cost-minimized energy systems designed for specific locales, such as district heating systems, both conventional and renewable local utilities production facilities, community energy storage systems, and even better managed electric vehicle charging station capacity, will offer concrete saving potentials in municipalities and smart city environments.
While reducing wasted electricity – and, therefore, saving significant expenditure on daily operating costs – businesses will be able to strengthen their brand image as truly sustainable commercial entities. In the end, I would argue that there is a significant win-win for businesses that want to remain competitive and to be seen as a trusted supplier. Digital twins are, of course, only a part of achieving a best possible decarbonized future but they will become an increasingly important one.
Are you looking for more ways to decarbonize your smart district? Find out here!
Our expert
Author
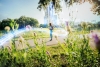
10 Steps to Finding your First AI Use Case
10 Steps to Finding your First AI Use Case
Listen to the blog here:
Artificial Intelligence, machine learning and data science seem inevitable to unlock the digital future. Do you want to jump on the bandwagon? Slow down, take a step back and ask yourself: Do you really know where to start? Does your idea really fit your organization? Sneak Peek: Failure is part of the process and concrete business value doesn’t come easy.
According to Gartner, by the end of 2024, 75% of enterprises will shift from piloting to operationalizing Artificial Intelligence (AI), driving a 5 times increase in streaming data and analytics infrastructures. Is your digital transformation journey about to begin? Great! However, getting started can be tricky. The big question is: how do you find a suitable AI use case and how do you know it’s the right one to kick off your digital journey? Our AI experts from Siemens Advanta identified 10 steps to find your first AI use case.
1. Select a real business problem
First off, don’t give in to the temptation of just using that cool technology you saw at a conference or of copying that fancy solution your competitor went for. Go for an ACTUAL challenge of your organization! This will help you build an ACTUAL project as opposed to a hobby: have a real “customer”, get a commitment, search for support, and a pull from the organization. Also, keep in mind that hobby projects are the first thing to be cancelled once operational pressure builds up. Working on a real pain point insulates your project from being stopped prematurely.
2. Define a clear “Why”
Before even looking at the data, there must be a shared understanding of the goal of the AI use case within the team. Merely “analyzing the data” is not going to work! The common understanding of what you want to achieve needs to be directly attached to the business problem: Go back to your organization’s strategy or major pain point and align potential use case outcomes (e.g., a strategic goal of a more bespoke marketing can be achieved by understanding different types of customers based on their purchasing behavior). New ways of ideation and innovation such as design thinking can be the right approach here.
3. Know your stakeholders
The healthcare industry holds one of the most significant investment opportunities for IoT technology ventures. We have observed its growth for years as investors keep leveraging better diagnostic and treatment ideas. The outbreak of the COVID-19 pandemic brought a lot more attention to the sector, and several digital health innovations emerged in 2020 in response to it. These IoT related solutions include applications that help with contact tracing, remote diagnostics and remote patient monitoring. We anticipate that the IoT new trends in this sector in 2020 will still be relevant in 2021, especially with the emerging need for vaccine cold chain management. These innovations will stay with us even beyond 2021 and will keep addressing various other health care challenges – supporting our ability to manage healthcare systems effectively.
4. Specify the data problem
Will we ever have an Artificial General Intelligence – an AI that can solve any problem, just like a human brain? This is highly disputed. Still, nobody contests the fact that today’s AIs perform well on specific, and sometimes very complex, problems. Make sure the selected challenge you want to tackle is defined in a way that is susceptible to AI. It has to be a data problem, such as:
- identifying anomalies
- grouping together entities that have similar features
- predicting outcomes
Formulate your problem as a data problem!
5. Get access to the right data
The data you use must fit the task, it must be sufficient in volume and quality and it needs to be accessible. If the data is not in shape, your use case will not get off the ground. Problems with the data can typically be fixed, but the fixes are all too often neither fast nor cheap. Processes might have to be changed and systems or IT landscapes adapted before you can get started. Plan significant effort to get the data in shape and ask the right questions early!
6. Calculate a business case
If you’re not in a research and development department, you are unlikely to get your AI use case funded or supported beyond a certain stage, unless there is a reasonable business case for the envisioned solution. This can be a daunting exercise. Estimating the potential benefits is already quite hard, determining the costs correctly is typically even more tricky (see our ROI whitepaper). If you manage to specify the benefits of your use case, you enable your whole organization to see the potential of the investment. For instance, increasing process efficiency or reducing manual efforts in your organization will provide you with the buy-in you need.
7. Prepare to fail fast …
Your digitalization journey is a learning journey – not only for you, but for most of the organizations out there. There are chances that your first use case might turn out differently as originally envisioned. Although total failure is a somewhat extreme scenario, partial rather than full success is the likely outcome. Be prepared to adapt! You might not end up with what you initially craved for, but when adapting learnings early on, your partial success might turn out to be exactly what your company needs.
Remember: failure is part of the process and concrete business value doesn’t come easy!
8. … but make something work
Since this is the first step in your digitalization journey, nobody expects a full-blown victory. Nevertheless, flat-out failure will not help you getting support for the next idea. Make sure to learn on the way so that you can either stop early or adjust. This will help you to give positive traction to digitalization efforts in your company and gather promoters to support you.
9. Start small …
Don’t let overwhelming organizational complexity hold you back in kicking-off your use case! Rather select a small and manageable subset, like a small region, a specific business unit, a selection of products or a particular type of customer. This will allow you to prove the value of your solution to the stakeholders while you learn about the important opportunities and threats at the same time.
10. … and be ready to scale
If your use case proves valuable, you don’t want to stay limited to this small subset! Ideally, your case can be swiftly adopted to be scaled to larger regions or more business units. This also means you need to balance efforts to standardize processes, document your initial steps and have productive infrastructure and operations in mind early so they can easily be applied to the expanded scope.
Huge potential to be leveraged with the right partner
For many business stakeholders, the journey of finding the first AI use case still needs to be demystified. Surely, there is no one-size-fits-all solution. The success of an AI project heavily relies on individual characteristics of your organization, its stakeholders and available data. As every journey is unique, it is important to start by answering the right questions early on and manage expectations. The potentials of Artificial Intelligence remain huge. However, often a trusted partner is needed to create transparency on the benefits of machine learning and other technologies for your business. Learn how we can leverage advanced analytics to create value here.
Our expert
Author
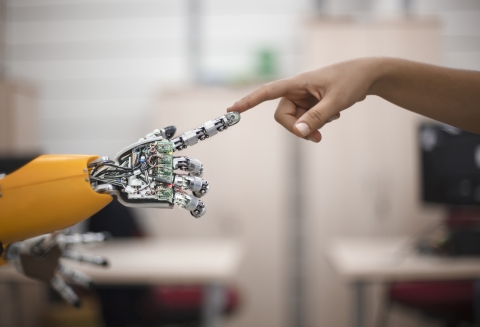